Artificial Intelligence (AI) encompasses the emulation of human-like intelligence in machines, enabling them to tackle tasks traditionally necessitating human cognitive abilities, including problem-solving and learning, reasoning, and understanding natural language. AI systems work through a combination of data, algorithms, and computing power. Here’s a simplified overview of how AI works:
The Birth of AI
The concept of AI dates back to ancient history, but it gained prominence in the mid-20th century with the birth of computer science. Pioneers like Alan Turing laid the foundation for AI by proposing the idea of machines that could mimic human intelligence. Over the years, AI has evolved from theoretical discussions to practical applications.
The Core Components of AI
AI systems rely on three fundamental components: data, algorithms, and computing power. Data serves as the fuel that powers AI, allowing machines to learn from past experiences. Algorithms are the sets of instructions that govern AI’s decision-making, while computing power provides the processing muscle for complex calculations.
How AI Learns and Adapts
One of the most remarkable aspects of AI is its ability to learn and adapt. Machine learning, a subset of AI, enables systems to analyze data, identify patterns, and improve their performance over time. This iterative learning process is what drives AI’s progress.
AI in Action: Real-World Applications
AI is already making waves in various industries. In healthcare, AI aids in disease diagnosis and drug discovery. In finance, it enhances fraud detection and trading strategies. Self-driving cars, virtual assistants, and recommendation systems are just a few examples of AI applications transforming our daily lives.
The Impact of AI on Various Industries
From agriculture to entertainment, AI is reshaping industries in profound ways. It increases efficiency, reduces costs, and unlocks new possibilities. However, it also raises questions about job displacement and the need for ethical guidelines.
AI Ethics and Concerns
As AI advances, ethical concerns emerge. Issues related to bias in algorithms, data privacy, and the potential for AI to outperform humans in decision-making are hot topics of discussion. Addressing these concerns is crucial for responsible AI development.
The Future of AI
The future of AI is limitless. Predictive analytics, personalized healthcare, and advanced robotics are just glimpses of what’s to come. AI will continue to push boundaries and redefine the possibilities of human-machine collaboration.
AI’s Role in Problem Solving
AI’s problem-solving capabilities are invaluable. It excels in solving complex problems that involve vast amounts of data and variables. This enables faster decision-making and empowers businesses to stay competitive.
AI and Natural Language Processing
Natural Language Processing (NLP) is a subset of AI that focuses on enabling machines to understand and interact with human language. It powers virtual assistants, language translation, and sentiment analysis.
Data Collection: AI systems rely on vast amounts of data. This data can be structured (e.g., databases) or unstructured (e.g., text, images, videos). The effectiveness of training AI models significantly relies on both the quality and quantity of data. learn more
Data Preprocessing: Raw data often needs to be cleaned, organized, and transformed into a format that AI algorithms can understand. This step may involve tasks like data cleaning, normalization, and this is feature of engineering.
The Algorithms Machine Learning : AI often employs machine learning algorithms to learn patterns and make predictions or decisions. Machine learning encompasses a wide array of algorithms, including:
The Supervised Learning: Models are trained on data with labels, where the input and the correct output are provided. The algorithm learns to map inputs to outputs.
Unsupervised Learning: Models get deeper insight into patterns and how to group related data points together from unlabeled data.
Reinforcement Learning: Agents acquire decision-making skills by engaging with an environment and receiving feedback through rewards or penalties.
Deep Learning: A specialized branch of machine learning employs multi-layered neural networks (known as deep neural networks) to process and extract insights from data. It excels in tasks that involve unstructured data such as images and text.
Training: During the training phase, the AI model is exposed to the data, and it adjusts its internal parameters to minimize errors or maximize rewards based on the type of learning algorithm being used. This process involves optimization techniques to find the best model parameters.
Testing and Evaluation: After training. To evaluate the performance of the AI model, it is tested on a different dataset (not used during training). Various metrics are used to evaluate how well the model generalizes to new, unseen data.
Deployment: Once the AI model performs satisfactorily in testing, it can be deployed in real-world applications. This could involve integrating the model into software systems, applications, or devices.
Feedback Loop: AI systems often have feedback loops where they continuously learn and adapt based on new data. This allows them to improve their performance over time.
Natural Language Processing (NLP): In the case of AI systems designed to understand and generate human language, like chatbots or language translation services, NLP techniques are used to process and analyze text or speech data.
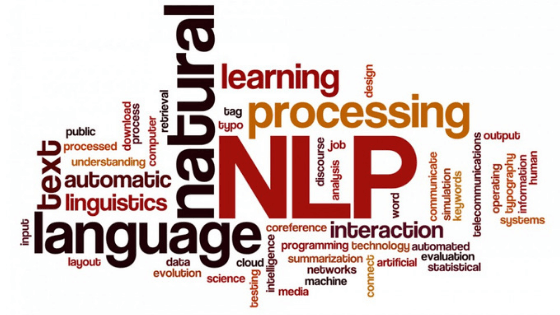
Computer Vision: For AI systems that work with images and videos, computer vision techniques are employed to interpret and extract information from visual data.
Optimization and Scalability: AI systems need to be optimized for efficiency and scalability to handle large volumes of data and real-time processing.
It’s important to note that AI is a broad field with various subfields, and the specific techniques and algorithms used can vary widely depending on the task and application. Additionally, AI is a rapidly evolving field, with ongoing research and development leading to advancements in AI capabilities.
How To Use Chat GPT – Read this also
Q1: What is Artificial Intelligence (AI)?
Q2: How does Artificial Intelligence work?
Q3: What are some real-world applications of Artificial Intelligence?
On Healthcare indrstry : AI helps with disease diagnosis, analyzing medical images, and drug discovery.
Finance: It’s employed for risk management, algorithmic trading, and fraud detection analysis.
Retail: AI drives chatbots for customer service, inventory control, and recommendation systems.
Autonomous vehicles use artificial intelligence for navigation and safety.
Customer Service: AI-driven Chatbots offer round-the-clock support and answer common queries.
Q4: Are there different types of Artificial Intelligence?
Narrow or Weak AI (ANI): This type of AI is designed for specific tasks and can’t perform tasks beyond its programming. Examples include incorporate digital helpers like Siri or Alexa.
General or Strong AI (AGI): AGI possesses human-like intelligence It is capable of carrying out every mental job that a human can. can. AGI is still theoretical and not fully realized.